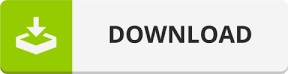
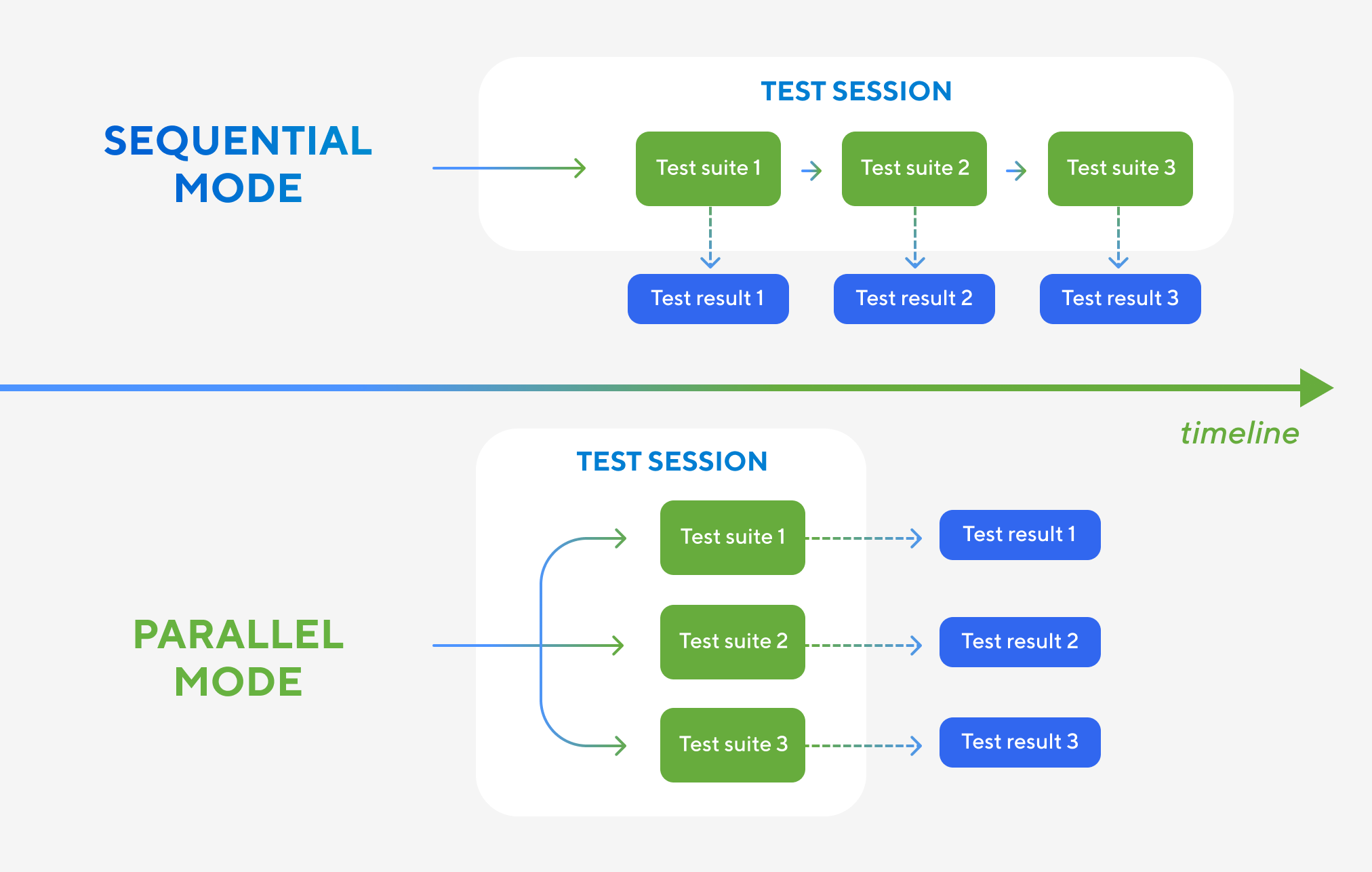
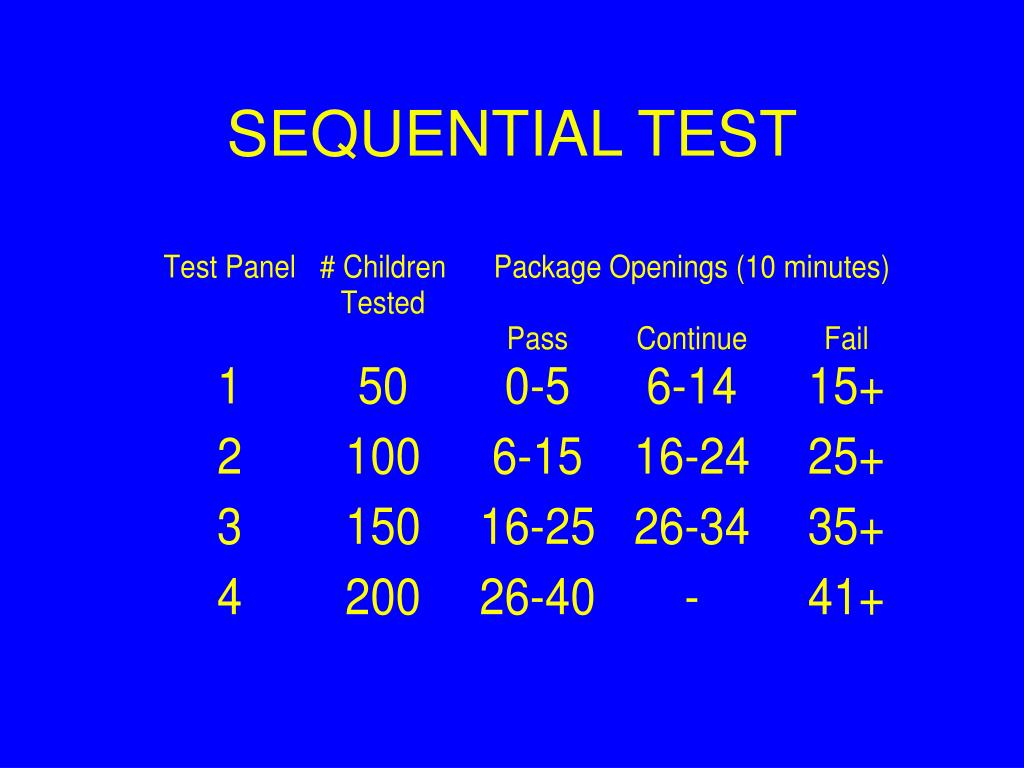
If we don’t find a significant difference, we proceed to collect another pair of observations and repeat the test. After collecting a new pair of observations from both groups, we apply our test. We use a z-test to see if there’s a significant difference between the treatment group and the control group in terms of, for example, minutes played on Spotify. For example, imagine that we’re running an experiment on 1,000 users split evenly into a control group and a treatment group. Peeking inflates the false positive rate because these nonsequential (standard) tests are applied repeatedly during the data collection phase. One of the most common sources of incorrect risk management in experimentation is often referred to as “peeking.” Most standard statistical tests - like z-tests or t-tests - are constructed in a way that limits the risks only if the tests are used after the data collection phase is over. Peeking is a common source of unintended risk inflation In Spotify’s Experimentation Platform, we’ve gone to great lengths to ensure that our experimenters can have complete trust that these risks will be managed as the experimenter intended. The intended false positive rate is often referred to as “alpha.” By properly designing the experiment, these rates can be controlled. In data science jargon, these mistakes are often called “false positives” and “false negatives.” The frequency at which these mistakes occur in repeated experimentation is the false positive or false negative rate.

Ultimately, this means limiting the risk of making poor product decisions.įrom a product decision perspective, the risks we face include shipping changes that don’t have a positive impact on the user experience, or missing out on shipping changes that do, in fact, lead to a better user experience. Spotify takes an evidence-driven approach to the product development cycle by having a scientific mindset in our experimentation practices. We can iterate faster and try new things to identify what changes resonate with our users.
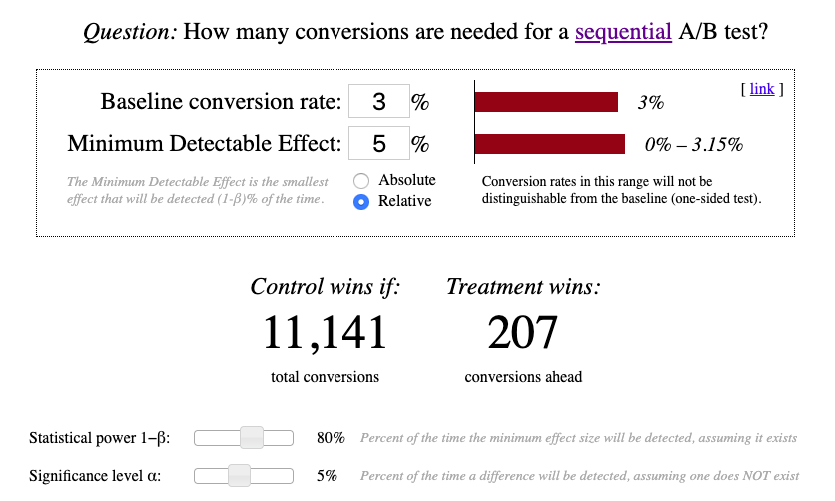
Solid experimentation practices ensure valid risk managementĮxperimentation lets us be bold in our ideas. We show that when you can estimate the maximum sample size that an experiment will reach, GST is the approach that gives you the highest power, regardless of whether your data is streamed or comes in batches.
SEQUENTIAL TESTING HOW TO
Even though the sequential testing literature is blooming, there is surprisingly little advice available (we have only found this blog post) on how to choose between the different sequential tests. The literature on sequential testing has developed quickly over the last 10 years, and it’s not always easy to determine which test is most suitable for the setup of your company - many of these tests are “optimal” in some sense, and most leading A/B testing companies have their own favorite. TL DR Sequential tests are the bread and butter for any company conducting online experiments.
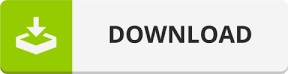